Available in Isatis.neo: an innovative way of classifying mineral resources
Posted on With the “Sampling Density Variance” tool, Isatis.neo offers an innovative methodology allowing robust resource classification, independent from sample number, block size, and domain extension.
In the mining industry, it is common to classify estimated resources as inferred, indicated or measured according to their confidence level which depends on the sample spacing and the expected production volumes.
This confidence level is classically derived either from the linear kriging variance or from a post-processing of conditional simulations. Both methods have their own limitations, the first one because it does not take into account data values (and the error distribution is rarely gaussian by nature), the second one because it is rather time-consuming and requires strong hypotheses. Isatis offers a third classification technique allowing the direct calculation of a Confidence Interval attached to the estimation of blocks in the framework of the Discrete Gaussian Model. Whilst producing CI’s faster than when resorting to conditional simulation, the mapping of confidence levels is less powerful than with simulations (where one can produce complex risk indices).
Whatever the method used, the confidence is computed for each individual block from the block model. The drawback is that resource categories depend on the size of the blocks and can vary quickly at small scale because of possible rapid variations from a block to the next one (which is often referred to as “the spotted dog syndrome”).
A post-process is then required to smooth categories. Another drawback is that the block support is not appropriate for long-term management implying larger production volumes.
Isatis proposes a new geostatistical classification of mineral resources which consists of two steps:
- Firstly, the spatial sampling density is characterized throughout the deposit using a specific volume quantity similar to the inverse of the classical density of sample points in space but taking into account the variographic structure of the grades of interest. The method requires the kriging at smu scale within “super” blocks with a size much larger than the actual block size and usually corresponding to specific production periods;
- Secondly, this volume is converted into a coefficient of variation corresponding to large production volumes, typically the expected annual volume to be mined. Then, a risk index is obtained by thresholding this coefficient of variation.
The examples below show and compare this risk index to classical classification on 2 cases:
- Copper grade with irregular sampling pattern – Courtesy Mines ParisTech/Codelco:
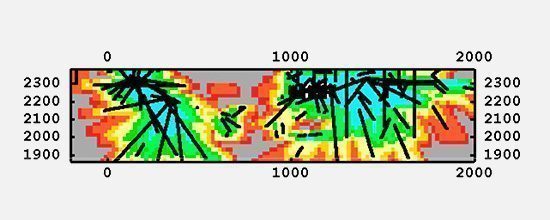
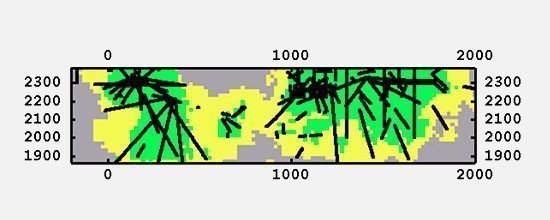
- Iron ore proportion with irregular sampling pattern – Courtesy Mines ParisTech/Vale:
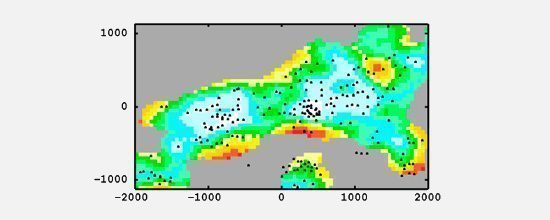
Why using this new index?
ADVANTAGES
- Robust classification independent from sample number, block size and domain extension
- Appropriate for mid and long-term resource management
- Coherent variation of the index in space
- Comparable index from one deposit to another
- Integrates uncertainties related to the sampling mesh and the spatial variability
BENEFITS
- Objective resource classification criteria
- Accessible index, quick and easy to get, does not require post-processing
- Simplified communication between teams
- Facilitated comparison of different deposits
REFERENCES
- A Specific Volume to Measure the Spatial Sampling of Deposits – Rivoirard J. & Renard D. (Centre de Géosciences, Mines ParisTech) – in Mathematical Geosciences – October 2016, Volume 48, Issue 7, pp 791–809
- From the Spatial Sampling of a Deposit to Mineral Resources Classification – Rivoirard J. and Al – Presented at Geostats2016, Valencia
Mining (14)
Nuclear Decommissioning (9)
Contaminated sites (7)
Oil & Gas (6)
Hydrogeology (5)
TAGS:
2D/3D (2)
Background images (1)
Big data (1)
Conditional simulations (7)
Contaminated sites (2)
Contamination (2)
Drill Hole Spacing Analysis DHSA (3)
Excavation (2)
Facies modeling (2)
Flow modeling (1)
Geological modeling (3)
Gestion des sites pollués (2)
H2020 INSIDER (1)
Horizon mapping (1)
Ice content evaluation (1)
Isatis (11)
Isatis.neo (16)
Kartotrak (8)
Machine Learning (2)
Mapping (3)
MIK (2)
Mineral resource estimation (7)
Monitoring network optimization (1)
MPS (2)
Ore Control (1)
Pareto (2)
Pollution (2)
Post-accidental situation (2)
Recoverable resource estimation (3)
Resource classification (2)
Resources workflow (1)
Resource workflow (3)
Risk analysis (3)
Sample clustering (1)
Sampling optimization (3)
Scripting procedures (3)
Simulation post-processing (1)
Site characterization (2)
Soil contamination mapping (4)
Time-to-Depth conversion (1)
Uncertainty analysis (2)
Uniform Conditioning (5)
Variography (2)
Volumes (2)
Water quality modeling (1)
AUTHORS:
David Barry (3)
Pedram Masoudi (2)
Yvon Desnoyers (2)
Pedro Correia (1)
Catherine BLEINES (1)
DATES:
2023 (2)
2022 (3)
2021 (2)
2020 (2)
2019 (8)
2018 (4)
2017 (3)
2016 (3)