Can you afford to bypass geostatistics for the sake of productivity?
Posted on Anyone involved with today’s mining industry understands that the sector is facing very tough challenges. And it is under enormous pressure from due cost control and budgetary management constraints that innovation and ingenuity must still find their way to propose new ways of tackling traditional issues. Mineral Resource Estimation (MRE) is no stranger to that conundrum and their practitioners all know the multi facets of the game: integrate more and more data, boost productivity, and come up with an answer fast and at the same time enriched with an assessment of the uncertainty that can be attached to that answer. All of that in a fraction of the time they used to have at their disposal and less resources to double check…
This is where we at Geovariances, believe that a little extra time and human resources devoted to the use of above standard geostatistical procedures will prove highly beneficial from an economic viewpoint in that context. The gains that can be had from optimizing the use of information and getting superior decision-making bases are gains you can bank on.
To fulfill this objective, Geovariances has been working for decades with the Geostatistics Research Group from the Paris School of Mines (Mines ParisTech) to bring advanced technologies and make them accessible to the mining industry.
These advanced technologies can be called upon in our geostatistics software package Isatis.neo.
They benefit your business in many aspects: cost-saving, time-saving, improved decision-making.
Multivariate geostatistics for improved resource estimation
Maximizing the use of existing information benefits each step of your estimation process and allows for decision-making optimization without spending to compensate for missing information.
It helps refine domaining by using a multivariate signature to flag domains.
It increases estimate precision when density plays a role and/or key variables have a high level of correlation and so, helps reduce ore/waste misallocation error.
This allows predicting the recovery functions on the secondary elements, deleterious or precious, when selection is made on a primary element.
Using multivariate conditional simulation, this helps optimize the process control parameters of mineral processing by reproducing the correlation between input variables (e.g. of gold and copper in a porphyry, grade and contaminants in an iron orebody; multivariate trace or deleterious elements for a coal product destined to metallurgical use, etc.).
Super Kriging for resource classification
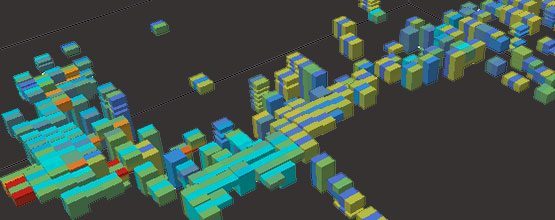
Also called Sampling Density Variance, this functionality has been available in Isatis for a couple of years and proves to be a really robust first pass approach to resource classification which truly captures the two key elements to consider: the density of information and the spatial variability of the grade(s) of interest.
This new classification methodology has several advantages. It provides a robust and coherent classification index independent from local fluctuations of arbitrary parameters by integrating the uncertainties related to the sampling mesh and the spatial variability. It is quick to calculate and facilitates the comparison of different deposits.
Super Kriging is particularly appropriate for mid and long-term resource management.
Conditional simulations for resource estimation and risk analysis
A platform of conditional simulation realizations, when valid as a plausible representation of the underlying reality, is an extraordinary conduit towards recoverable resource estimation. It is in certain cases almost the only one providing a practical solution, for example when the grade variables that are used for making ore/waste allocation decisions are non-additive but are in fact ratios of additive variables (variable density and variable metal quantity for ex.). Resorting to conditional simulation will prove efficient as each realization can address varying support effect or information effect issues or the non-additivity of the grade variable (by co-simulating the two input additive variables).
And in fact, conditional simulations offer the advantage of providing the adapted platform to characterize the risk attached to the recoverable resources.
Indeed, all simulation realizations end up in a post-processing phase to calculate the distribution of possible grade values for each block (and associated min, max, mean, quantiles) and characterize the resource variability when applying production constraints (cutoff, block size, grade control…). This allows the inference of optimistic and pessimistic scenarios for a given confidence interval and the probability for the grade of a block to exceed a cutoff.
Conditional simulations and Simulation Reduction to characterize the risk attached to a project due to the uncertainty on the resource
A key property of geostatistical conditional simulation is that a family of images of the orebody that presents a range of plausible possibilities is produced, each reproducing the histogram, variogram of, as well as honoring the input data.
The question could arise then of how to derive relevant information from this vast array of possibilities in an efficient manner.
Indeed, even if the production and post-processing of multiple conditional simulations realizations are now more than ever a practical solution to many MRE problems, the ability to still focus on a select few realizations remains very appealing to all practitioners.
The objective of the Simulation Reduction approach is to be able to evaluate projects using a representative subset of simulations selected among a larger set, each realization being associated with a probability of occurrence.
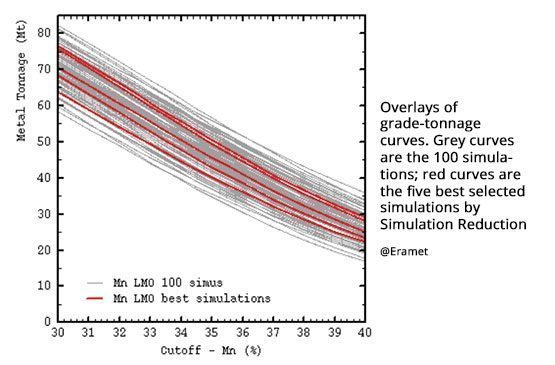
This approach is easy to set up in an industrial context. As an example, Simulation Reduction has been applied to the Tiébaghi orebody at SLN. Five sets of simulated recoverable grades have been picked up by the procedure (with probabilities of occurrence of 70%, 20%, and about a few percent). Given the more manageable number of realizations, a characterization of resource uncertainties has been made possible. From these five representative scenarios, SLN has derived five pit designs allowing the optimization of the entry sectors for the mining operations.
Download the technical article →
However promising conditional simulations are, they are not widely implemented because they are often found too time-consuming … Once again, Geovariances is working on a solution
Despite this inherent ability of simulation to tackle risk analysis, a series of factors have prevented their incorporation into the mainstream chain of information processing prevalent in MRE. The factors that have impeded simulation’s progress vary in nature from a series of cultural or regulatory blocks to more technical elements. The main technical block has been that of performance: it still takes a long time to produce simulation realizations in sufficient numbers to allow meaningful risk analysis to be performed. A problem made worse when the size of the dataset increases as tends to be the case nowadays with the emphasis put on optimizing the use of all available data.
Geovariances has been managing (in partnership with the Center for Geostatistics from Mines ParisTech and 6 industrial sponsors) a specific R&D consortium (SPDE) meant to develop a new engine for conditional simulation production adapted to the specific context of MRE that will offer a game change in performance (drastic reduction of time required to produce large numbers of realizations, using large numbers of data).
In that context it is worth remembering the Vision of one of the historical giants in Geostatistics, Dr. André Journel who almost 15 years ago was writing (Journel and Kyriakidis, 2004):
“Now that computer hardware and (increasingly) software is not anymore an issue, our hope is that the mining industry will catch-up with other engineering fields and capitalize on the general concept of virtual simulation of any important mining plan decision, whether at the early stage of a mine planning and resource/reserve evaluation or at the later stage of grade control.”
Mining (14)
Nuclear Decommissioning (9)
Contaminated sites (7)
Oil & Gas (6)
Hydrogeology (5)
TAGS:
2D/3D (2)
Background images (1)
Big data (1)
Conditional simulations (7)
Contaminated sites (2)
Contamination (2)
Drill Hole Spacing Analysis DHSA (3)
Excavation (2)
Facies modeling (2)
Flow modeling (1)
Geological modeling (3)
Gestion des sites pollués (2)
H2020 INSIDER (1)
Horizon mapping (1)
Ice content evaluation (1)
Isatis (11)
Isatis.neo (16)
Kartotrak (8)
Machine Learning (2)
Mapping (3)
MIK (2)
Mineral resource estimation (7)
Monitoring network optimization (1)
MPS (2)
Ore Control (1)
Pareto (2)
Pollution (2)
Post-accidental situation (2)
Recoverable resource estimation (3)
Resource classification (2)
Resources workflow (1)
Resource workflow (3)
Risk analysis (3)
Sample clustering (1)
Sampling optimization (3)
Scripting procedures (3)
Simulation post-processing (1)
Site characterization (2)
Soil contamination mapping (4)
Time-to-Depth conversion (1)
Uncertainty analysis (2)
Uniform Conditioning (5)
Variography (2)
Volumes (2)
Water quality modeling (1)
AUTHORS:
David Barry (3)
Pedram Masoudi (2)
Yvon Desnoyers (2)
Pedro Correia (1)
Catherine BLEINES (1)
DATES:
2023 (2)
2022 (3)
2021 (2)
2020 (2)
2019 (8)
2018 (4)
2017 (3)
2016 (3)